Data Science vs Data Analytics
In this age of big data, the terms “Data Science” and “Data Analytics” are often used interchangeably, which leads to confusion regarding their roles and contributions. However, they have clearly different characteristics and roles in the world of data. The main aim of this article is to through light on their similarities, differences, and how they complement each other. We’ll explore the exciting world of Data Science and Data Analytics and will highlight their similarities and differences to shed light on their roles in exploiting the power of data. Consider it an overview of the world of data analysis.
The Dance of Data
Imagine a big ballroom filled with data from different sources, including structured and unstructured data. In this digital masquerade, Data Science and Data Analytics step onto the dance floor as the lead performers, each with its own dance moves. Data Science uses complex algorithms and models to analyze and interpret the data, while Data Analytics uses statistical methods and visualizations to make sense of the information. Together, they create a beautiful dance of insights and discoveries that can help organizations make informed decisions and improve their performance
Defining the Terms
Let’s start by defining these terms:
What is Data Science?
Data Science is a broad field that uses scientific methods and tools to extract useful information and insights from data. It involves several stages, including data preparation, data exploration, data modelling, and data visualization. Data Scientists use machine learning algorithms, statistical analysis, and data visualization tools to reveal hidden patterns, make predictions, and inform decision-making.
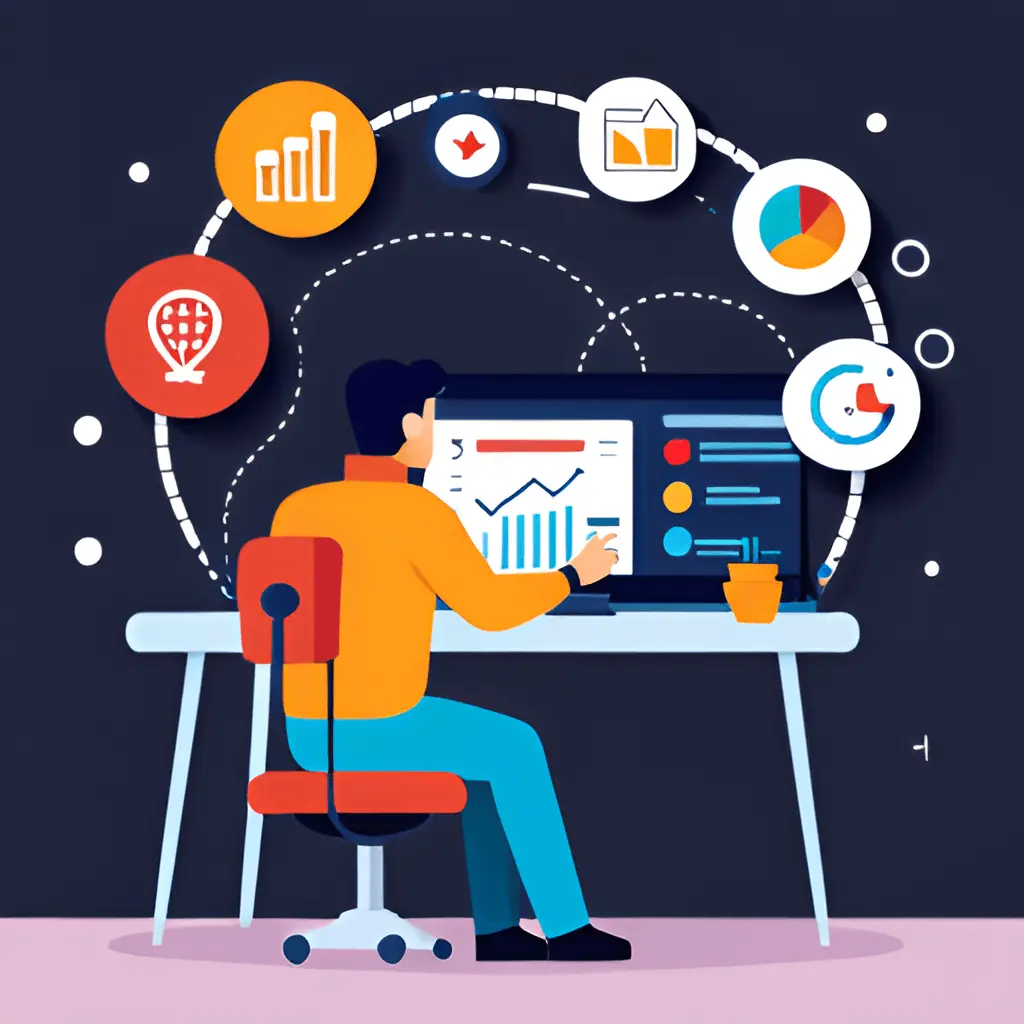
What is Data Analytics?
Data analytics is the process of examining data to find useful information and draw conclusions. It involves collecting data, cleaning and organizing it, analyzing it using statistical techniques and interpreting and communicating the results. The goal is to discover patterns, trends, and insights that can inform decisions and actions. Key steps include asking the right questions, preparing the data, applying analytics methods, and presenting meaningful information. Overall, data analytics converts raw data into actionable knowledge.

Data Science vs Data Analytics, similarities
Both fields deal with data and require a strong knowledge of mathematics and statistics. They also require proficiency in programming languages like Python or R for manipulating and analyzing data. Here are some commonalities:
Data Science | Data Analytics |
---|---|
Deals with large volumes of data | Deals with large volumes of data |
Requires knowledge of statistics and mathematics | Requires knowledge of statistics and mathematics |
Uses programming languages like Python or R | Uses programming languages like Python or R |
Aims to extract insights from data | Aims to extract insights from data |
Data Science vs Data Analytics, Differences
While there are similarities, the two fields have different focuses. Data Science is more about predicting the future based on past patterns while Data Analytics focuses on drawing conclusions from raw data. Here are some key differences:
Property | Data Science | Data Analytics |
---|---|---|
Objective | Explore unknown, find patterns and trends | Answer specific questions, solve defined problems |
Depth | Dives deep into data, complex algorithms and models | Stays close to surface, descriptive stats and visualization |
Scope | Long-term, exploratory projects | Focused, tactical projects to answer business questions |
Methods | Machine learning, advanced statistical models | Statistical analysis, SQL, data visualization |
Data Sources | Unstructured and structured data | Mainly structured data |
Output | Predictions, recommendations | Insights based on current data |
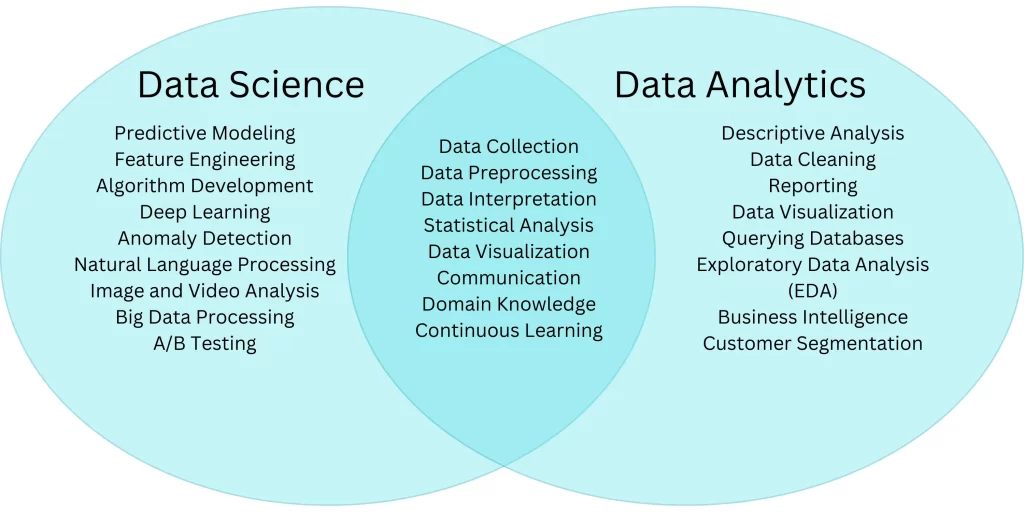
Conclusions
In the big room of information, both Data Science and Data Analytics are important dancers. They each have their own special moves and styles, which help create a beautiful performance of insights from data. Data Science looks at the details of the data and finds hidden patterns, while Data Analytics makes sure that decisions are made quickly and accurately.
So, when you hear these terms, remember that Data Science is like a magician who shows you things that are hidden, and Data Analytics is like a choreographer (Dance producer) who makes sure everything flows smoothly. Together, they make sure that the performance of data continues to be amazing and informative.
Also, Read Types of Artificial Intelligence